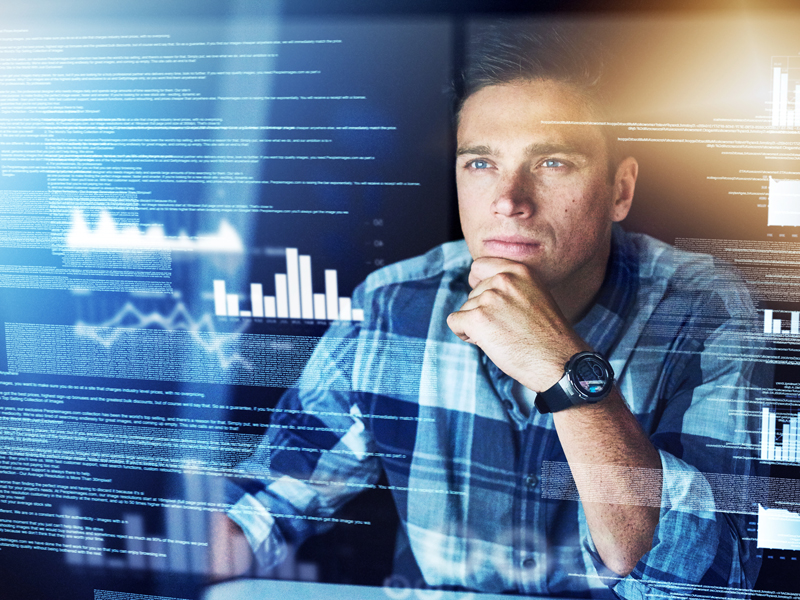
Top 5
To measure and manage your risk, you need to do three things well: define and quantify a company’s financial distress; understand the time horizon of the risks you’re taking; and understand the drivers of potential default. At Kamakura Corporation, we are strong advocates of using quantitative tools to answer these questions. Specifically, we use reduced form models, which provide easy visualisation of everything you need to know.
Kamakura’s Risk Information Services monitor a global index that contains over 38,000 businesses spread across 62 countries to analyse default probability reports and other financial predictions
Financial distress is defined as the elevated probability that a firm will fail to meet its financial obligations, but elevated probability isn’t a very helpful measure when you’re assuming some of the risk. To quantify the distress level, you can use a variety of tools, including fundamental research, credit scores and reduced form models.
Many of these tools have their limitations. Credit scores have an ambiguous time frame and do not answer the question of what is driving the default risk. Forecasting introduces biases. Financial information, while useful, is backwards-looking.
Looking forwards
When you’re driving, you use your rear-view mirror frequently, but you spend most of your time looking through the windshield. Identifying and quantifying financial distress should be a similar process. That means you need a tool that can incorporate history, while also focusing on forward-looking factors. Reduced form models, like Kamakura’s Risk Information Services (KRIS), allow you to do this. KRIS can be used to monitor a global index that contains in excess of 39,000 businesses spread across 68 countries. With such a wealth of data to draw from, it is easy to analyse default probability reports and other financial predictions.
Risk managers, investors and financial experts can then examine this sophisticated analysis as a key part of their decision-making process. It is, therefore, an essential tool in understanding which businesses to support and which ones are too risky to back. Crucially, a reduced form model like KRIS is sophisticated enough to incorporate a complete set of inputs and can provide a probability of default that corresponds to specific time frames.
Logistic regression models allow the user to calculate the most accurate predictions of default probabilities for a given set of inputs. The Kamakura models use company-specific information as well as macro variables (company accounting and market-based variables, along with interest rates and indicators of macroeconomic conditions) to deliver a default probability that can be used to directly assess a company’s risk. Further, a nested family of logistic regressions produces a highly accurate term structure of default.
Our current version of KRIS is version 6.0. This version benefits from the addition of nearly 500,000 observations compared with the previous database. The addition of more than five years worth of incremental data in the aftermath of the credit crisis has dramatically enhanced the insights that our models bring to users. Observed company failures, for instance, are 31 percent higher than with our previous model.
The credit crisis that occurred between 2006 and 2010 was the most severe crash to occur during the span of the Kamakura modelling database; the data shows that financial institutions and other highly leveraged firms were the most vulnerable in a crisis. Moreover, the search for yield over the past years has led many investors to high-yield investments and longer durations, which necessitate improved risk management practices by portfolio managers. We encourage our clients to utilise KRIS as much as possible so they can understand how reduced form model tools can help them to evaluate historical inputs and forward-looking inputs, and their various correlations to default risk. You can also analyse all these variables within a specific time horizon.
Finding fault
Before you perform any in-depth analysis, it is helpful to know which firms have already been identified as having an elevated probability of failing to meet their financial obligations. At Kamakura, we use a ‘troubled company index’, which shows the percentage of 39,000 public firms with a default probability of more than one percent. An increase in the index reflects declining credit quality, while a decrease reflects improving credit quality.
Of the firms in our coverage universe, 10.63 percent had a one-month default probability of more than one percent as of April 3, 2018. As credit managers, we want to be forward-looking. We use analytics to create an expected cumulative default rate, and we have further refined the list to focus on the rated companies in our coverage universe. Interestingly, our analysis shows that while the risk of financial distress in the short term has been low and stable, the default risk in the five-to-seven-year period has been growing.
Defaults can occur for any number of reasons and certainly aren’t limited to young, inexperienced firms. Aggressive expansion leading to financial instability is a common cause, as is relying on revenue streams from volatile, rapidly changing industries. Poor management at boardroom level can also be a factor, while macroeconomics issues beyond a company’s control can push organisations towards the edge. Although the reasons behind a default may be varied and difficult to pin down, data analysis will usually reveal some telltale signs.
Using Kamakura’s default probability solution, investors and creditors can assess a variety of different factors to achieve a predictive power that is unmatched anywhere else in the market. Transparency, of course, is paramount, so we ensure that nothing is withheld from our clients. This allows our service to be as comprehensive as possible, while still complying with all the credit modelling requirements stipulated under the Basel II provisions of the New Basel Capital Accord.
Company-specific risk
Corporate credit managers and traders may focus on shorter-term risks, while lenders and investors are more concerned with the longer-term risks of a counter-party. The time horizon that is most relevant will vary with every business and every lending or investing situation. Therefore, not only do you need to define the most relevant time horizon, you need the ability to measure the risk and the drivers of the risk over that period.
We will use the Noble Group as an example (see Fig 1). Noble is a commodities trader and was once one of the largest in Asia, but the firm defaulted on its obligations in March. The graph makes it clear that this should not have been a surprise: notice how the quantitative default probabilities show an increasing risk of default for the company in both the one-month and the one-year categories. You can see that in May 2017, both default probabilities jump up, and the expectation of default within the year begins to be significantly higher than the probability of default within one month. Given the announcement by the firm last month, this analysis proved to be very accurate.
The second thing to know when dealing with distressed firms is what is driving the default probabilities. KRIS reveals the drivers of default. In our Noble Group example, net income, oil prices, market volatility and the company’s stock volatility combined to drive the increased risk. KRIS combines both forward and backwards-looking factors, allowing us to quickly understand what needs to be monitored, including the absolute and relative levels of default probability.
You need this information to make an informed decision about a distressed company. It helps you determine whether to take the risk of extending credit or to pursue a range of alternative options, such as avoiding the risk, shorting the risk or hedging the risk. As Professors Robert Jarrow and Arkadev Chatterjea wrote in their book An Introduction to Derivative Securities, Financial Markets and Risk Management: “We live in an imperfect world, in which it is hard to find perfect hedges.”
One of the goals of a risk management system is to answer the question: “What is the hedge?” If there were a single source of risk and a single measurement to quantify it, the answer would be easy, but of course, that’s never the case. The strength of the Kamakura approach is that we offer an integrated measure of all the risks and their sources, with a definitive time horizon for each. Finally, you cannot consider the time horizon without addressing the debate regarding ‘point-in-time’ and ‘through-the-cycle’ credit indicators.
The reason for the debate is the vagueness of the maturity and default probabilities for a specific company at that point in time. When you use a quantitative default probability, the debate becomes meaningless as the default probability has an explicit maturity with a defined meaning. All default probabilities that exist today at all maturities are point-in-time default probabilities, while the longest maturity default probability for a given firm is the through-the-cycle default probability. When you’re dealing with a distressed company, you can’t afford to leave any stone unturned.